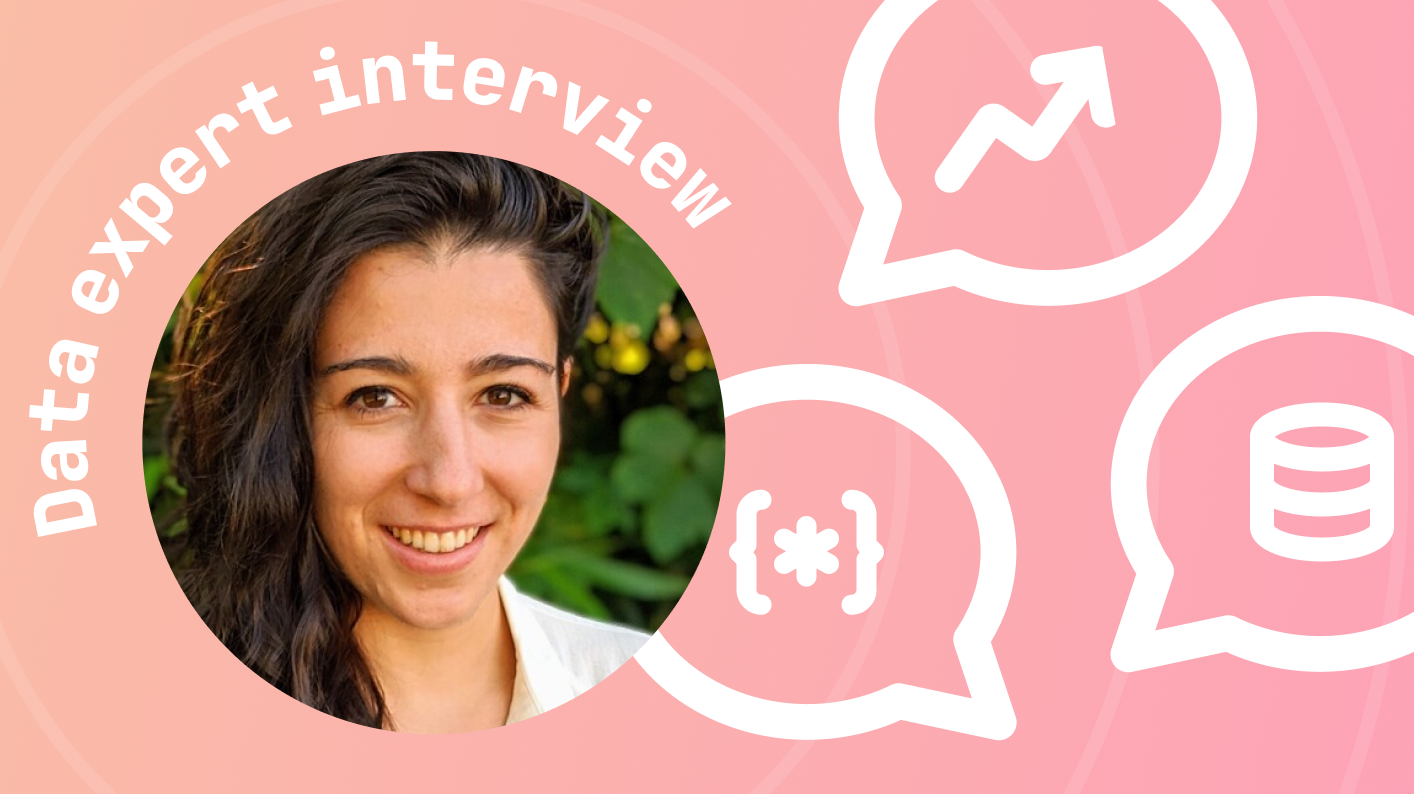
Recently, I sat down with Sarah Krasnik Bedell, Growth Lead @ Prefect to learn from her data expertise. During the interview, Sarah dove into her career journey so far, advice for closing the loop between data and business impact, an unpopular data opinion, and much more…
First off, tell us about your journey to data engineering and growth marketing…
What interests led you to data?
My entry into data was from a math background; I like tables, organization, and a reason for doing things. At first, I got into data science, but I quickly realized how much data engineering you need to do first - there's a lot of work to organize, clean, transform, and understand data before you can actually make any sort of impact.
The experience was similar to driving a car and looking under the hood to understand how it all works together so you can fix it. Understanding the importance of how things work and how to fix them led me to data engineering.
As the Growth Lead, what are you responsible for?
My responsibilities combine marketing and product growth for our commercial offering, Prefect Cloud, to help us understand how to make the product even more intuitive and helpful. This combines growth marketing and broader marketing (such as brand performance, paid, and product marketing) to help us improve how we explain Prefect to the world and bring more people into our ecosystem.
Think about growth as acquisition → activation → monetization:
We start with people’s exposure to Prefect, then their ability to be successful with the product as it exists today, and the last piece is the self-service side of the business to make sure people understand the value created and we continue to make it more valuable.
Both the growth and marketing sides of my role involve uncovering information to help us focus. For a marketing example, we have implemented front-end analytics to help us understand where people are most interested on our website and documentation as well as how we can make the product experience the most personalized and helpful. These findings and initiatives play a big part in our strategy to help us prioritize activities, such as interesting blog topics.
"Think about growth as acquisition → activation → monetization."
What’s your biggest piece of advice to someone else in your role?
Perfect is a very technical product, so my first advice is geared toward someone doing growth and marketing at a developer tool company:
You have to understand and empathize with your users. My background in data engineering helps me understand the core concepts, take things for a spin, and understand our user persona. I switched to focus on developer tools because I was a heavy user of many different data engineering and analytics tools and I really empathize with the persona.
Thinking more broadly for someone in growth and marketing:
Data is a very interesting point, but always has to live in context. It’s very important to find the balance between the context that you're living in and things that may be harder to quantify with the actual quantitative things that you see. You have to balance them together for the complete picture.
"Data is a very interesting point, but always has to live in context."
What’s your favorite non-Omni data tool?
Especially lately, I’m a big proponent of the operational analytics landscape where tools like Census and Hightouch live. To me, this space is a delight to use because these tools usually target less technical users so they’re very much plug-and-play. As long as you have credentials, you can move data from your warehouse to another tool. The combination of using Amplitude’s event data with the more static data in our warehouse yields a lot of interesting automated insights we can use to help people.
Now, let’s dive more into data at Prefect…
What are the key components of your data stack?
From a high level, we use:
Google Cloud BigQuery stores data from our various SaaS tools (such as Stripe, Salesforce, and Amplitude) and we are moving towards Omni as our business intelligence layer.
However, something that excites me most is that we bring this full circle and pipe information back into our other tools. For example, we use Customer.io and this data helps drive our email strategy both to get new features in front of our audience as well as docs that will be most helpful to different users at different times.
We bring everything together to help people in a more automated way.
What data challenges or goals are top of mind for you right now?
"...Our biggest advantage might also be our biggest challenge: we are a data company."
Self-serve analytics can be very hard depending on where you work and the backgrounds of stakeholders; how much they care or think about data has an impact. At Prefect, we have a lot of people who are very data literate and it’s natural for everyone to think about data. However, our biggest advantage might also be our biggest challenge: we are a data company.
Because everyone is so hungry for data, we prioritize self-service over structure and we empower people to answer their own questions. We’ve done a great job of building out our most important KPIs so we understand what we need to run the business, but one of my goals is to formalize the process for going deeper into the nuts and bolts. Not only do we want to have a high-level understanding of each functional area, but get deep into answering the questions surrounding why things happen, not limited to what happens.
What’s a common challenge when working with stakeholders, what’s your advice for improvement?
Previously, before I worked at a data company, the biggest challenge was getting people to think critically about data and not just trust what they see.
My least favorite phrase is “Something looks off” - why does it look ‘off’? What does ‘off’ or ‘on’ mean? While there is intuition behind that, there’s a lot more critical thinking to identify whether it’s a business problem (“Oh no! Orders are down!”) or a data problem (“That doesn’t match what I know happened, perhaps something is broken?”).
It’s important for stakeholders to think about ‘off’ critically so it can go into the right bucket for action.
What stands out to you about using Omni so far?
Now as more of a stakeholder (and not the person producing the data), I still want to be able to quickly answer my own questions. Especially if it’s just a one-off question, I don’t need it to be the most complex query and I don’t want to bother an engineer. I love the ability to write a SQL query and visualize something really quickly in Omni; it’s fantastic.
Previously, if I wanted to do this I would write a query in BigQuery, download the data, and put it in something to visualize it (dare I say, ‘Google Sheets’?). The process was tedious with a lot of copy and paste. I’d much rather utilize the modeling we’ve already done and not have to replicate anything - even if it’s not something I am going to come back to because there are a lot of those one-off things that actually come up!
Being able to answer questions and model things that you aren’t going to come back to is actually often more important than the things you don’t come back to because those things can come up over and over again and end up taking a lot of time. Now, the workflow in Omni really supports our self-serve model.
"I love the ability to write a SQL query and visualize something really quickly in Omni; it's fantastic."
What’s your unpopular data opinion?
Recently, I’ve been thinking a lot about identity resolution which is very top of mind because we have a lot of cross-domain traffic (our website, product app, etc.) we need to understand.
I think data people often have tunnel vision in terms of ‘this is the way to do this’ or ‘these are the tools to use;’ they’re trying to think broadly by looking at all the data. You do need to look at the data, but when I was a data engineer I spent a lot of time looking backward and working with engineering to ask ‘is this good data?’ and ‘how do we structure the product to generate data that’s conducive for analytics?’.
I believe if data people stop themselves from only thinking about ‘What I can do NOW?’ and accepting when bad data is created, there’s an opportunity to think about it more productively by thinking critically about what goes into producing good data.
What didn’t I ask you?
It’s important for folks to think about different aspects of data and their data team. I think it’s important to think about these parts: data generation, data consumption, and data activation. There are discrete things you need to focus on in each of these camps:
- Data generation: Thinks about how data is created and engages with engineering to understand the core parts of their product and how it’s implemented.
- Data consumption: Thinks about how to model data, who to expose it to, how to visualize it.
- Data activation: Thinks about what you’re doing with your data. You can’t just rely on the data consumption piece or you’ll just fall flat in the water.
All of these should come together to ultimately help you gain insight, automate, and drive value.
Thanks so much, Sarah!
For more tips and hot takes from Sarah, be sure to follow Sarah's Newsletter.