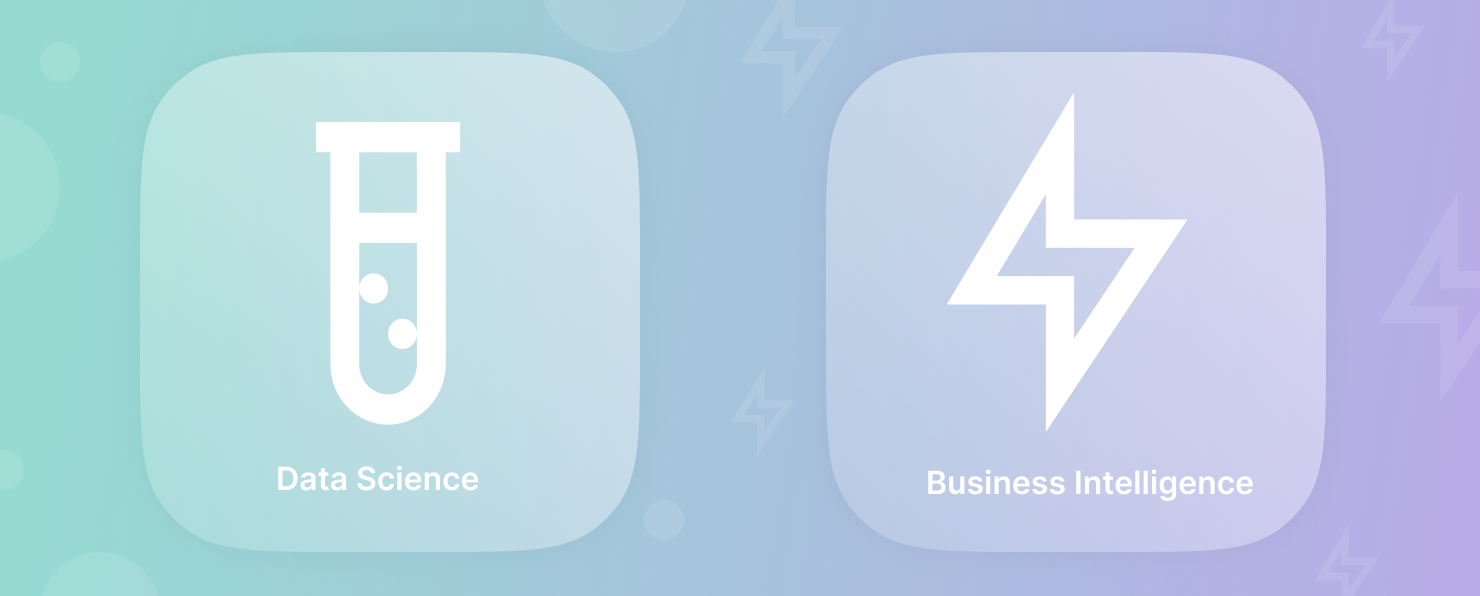
What is the relationship between data science and business intelligence? #
Over the years I have been asked this question many times, and of course the answer is ‘it depends.’ It depends on the maturity, size and needs of your organization. The relationship changes as roles and technology changes within your company.
TLDR: Data analysts own the swim lanes for data in your organization -- data management, reporting, the later stages of data transformation (though getting earlier and earlier as analysts get more technical), and day-to-day decision making with data. Data scientists focus on stepwise improvements that integrate data into improving the business, things like research and core product development.
I had a little fun and asked chatGPT this question, and it gave a pretty good, encyclopedic answer –> “Data science and business intelligence (BI) are related fields that both involve using data to make informed decisions.
Business intelligence (BI) is a set of tools, techniques, and processes that help organizations make better decisions by providing them with relevant and actionable data. Data analysts are responsible for selecting and implementing these tools, as well as maintaining and updating them. They also define data definitions and are responsible for general reporting.
Data science is a broader field that encompasses techniques such as machine learning, statistical analysis, and data visualization. Data scientists are responsible for conducting research and performing ad-hoc analysis to uncover insights that may not be immediately obvious. They often have a longer time horizon and less internal interaction compared to data analysts.
Both data science and BI are important for making data-driven decisions and can be used together to gain a more complete understanding of a business's operations and performance. Data scientists can inform the development of BI systems, and data from BI systems can be used as input for data science models.”
The deeper answer to this question heavily relates to how your organization is set up. #
In most mature businesses, data science and analytics are pretty distinct functions. Data scientists are working on algorithms and research, often with much longer time horizons and less internal interaction. They are nearly always a centralized team. Analyst teams can be centralized, but also can sit in lines of business, with cross-functional responsibilities and P&L alignment (for example in marketing).
In this case, the ownership is obvious; analytics teams are using and best equipped to select and own your BI tools. Data science teams will certainly have input into the selection process (and be a valuable resource for defining metrics) just as the marketing or engineering team might, but this is one of the crucial jobs of your analyst team.
In terms of working together, the structures vary widely. As I mentioned above, many times data science is given space to focus on longer term projects so involvement in day-to-day reporting and metrics may be non-existent. In other organizations, data science can be a mentorship path to the analyst team and the science team may have the most seasoned analysts as well. In this case, the data science org will have lots of impact on metric definitions and the BI toolkit.
In the case of a smaller org, the lines get more blurry. Your first data hire may identify as a data scientist or a data analyst. That said, early data work should be heavily focused on analytics (walk before you run). In this case, your data science team may be your analytics team and tool selection and ownership would live inside your hybrid data org.
What about analytics engineers? The biggest role change over the last decade is the addition of the analytics engineer role. An analytics engineer specializes in the design, development, and maintenance of data analytics systems. They work to ensure that data is easily accessible, accurate, and reliable for data analysts, data scientists, and other users. This role is a continued signal to the importance of data and data quality in organizations, and the need for more people with diverse skills to handle the amount of data and data prep data environments.
Ultimately data science, data analytics, and analytics engineering titles get thrown around for similar responsibilities, and the lines continue to blur. No matter your data teams’ titles, get your analytics in order before you start hoping for magic from your data science team.